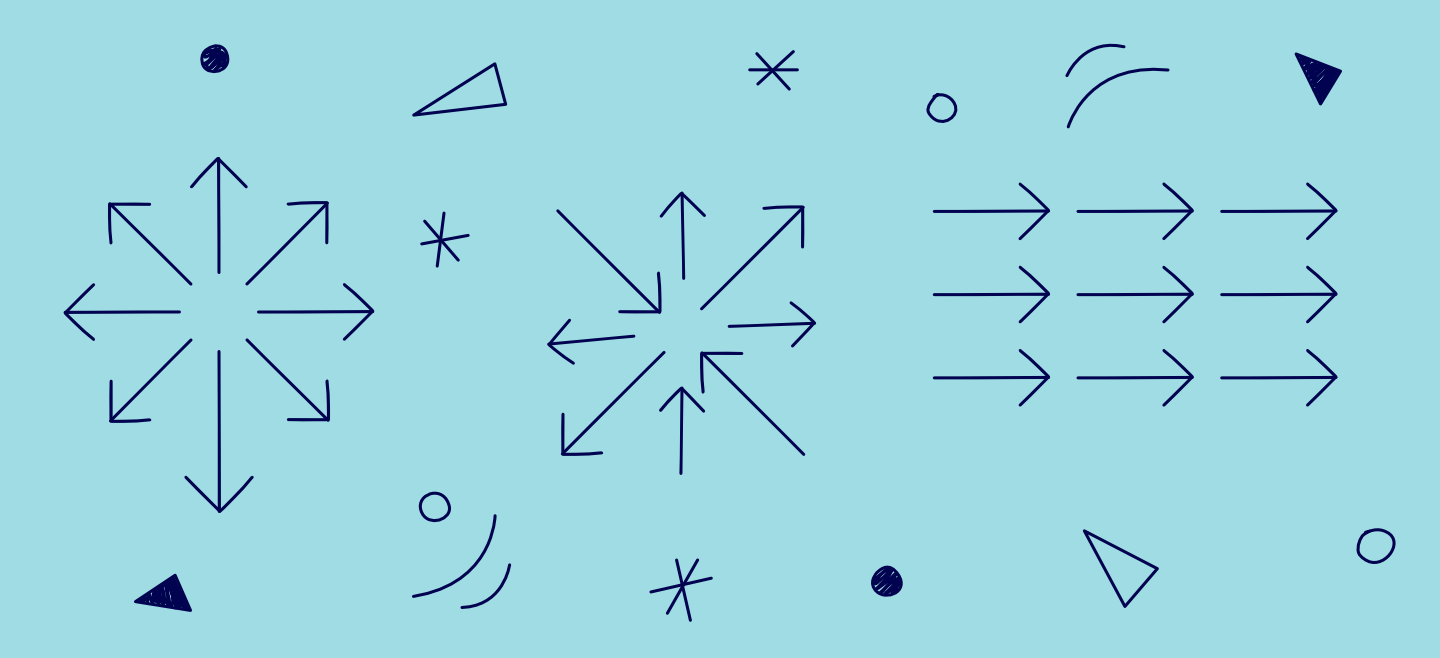
Refocus key priorities: from Tech to Eco-system
The third pillar of this manifest builds upon the concept of Relevant Data and the redefinition of algorithms as impact-creating machines. But above all this third pillar lives up to one of the most important lessons learned from earlier tech-driven disruptions; in order to make the most of new technology one shouldn’t focus on more technology.
As data derives its relevance from being linked to a clear objective and an algorithm becomes trustworthy on the basis of the impact it creates in its larger business context, we must refocus our priorities from tech to eco-system. In the 2020’s it is obvious we need an eco-system with tailor-made institutional and contextual (non-tech) circumstances that enable data and algorithms to live up to their potential.
‘Data and algorithms can only add real value and drive positive change when they are part of an trust evoking eco-system that is fit for purpose.’
To install a supportive eco-system this manifest identifies three key priorities:
- Ethical inclusiveness
- Updated laws and regulations
- Thought leadership in economics and business
Ethical inclusiveness
Ethics are about shared human values that underlie our human behaviour. We need to create a common ground to make shared human values an integrated part of data and algorithm driven innovation. If we don’t do it right now we end up with dazzling innovations that the majority of us ultimately doesn’t want. This will not only dramatically slow down future innovations. It will also be killing for the trustworthiness of data and algorithms in general.
‘Tech driven sense making is useless. We need ethical inclusiveness to make data and algorithm driven innovations acceptable from a human perspective.’
To apply ethical inclusiveness in the core of a corporate digital twin this manifest introduces three human centred ethical principles:
We seize opportunities to the max
To keep our world liveable and find answers for today’s mayor challenges we owe it to next generations and ourselves to make the most of data and algorithm driven innovation. Exploring the unknown is the only viable way forward as easy solutions no longer exist and hanging on to the status quo is no option.
We don’t accept moral haze
We are honest about why and how corporate digital twins perform. This means we can explain why particular choices (in design, implementation or use) have been made or why certain (combinations of) human values are considered more important or meaningful compared to others.
We pursue data and algorithm integrity
We acknowledge that data and algorithm integrity is a ‘conditio sine qua non’ for the trustworthiness of a corporate digital twin. On-going investments in raising the accuracy, consistency and reliability of data and algorithms are the standard. And when things don’t go the way we would have wanted, we’ll be transparent and responsive.
Updated laws and regulations
Laws and regulations are vitally important to define boundaries and rules of engagement in an eco-system. However, for data and algorithms there are many legal topics, issues and dilemmas that have yet to be fully addressed by our legislative systems. And to make things worse, most of these systems are not built to keep up with the speed of tech revolutions.
‘What’s the liability status of a private sector algorithm, that uses multi-sensor private and public data, and is designed to have impact in the public domain?’
The three most urgent regulatory domains that require updating are:
Data ownership and -usage
We must enforce by law that data is a new and independent factor of production. It should receive the same legal status as land, labour and capital. In designing a legislative framework for data-ownership the unique interrelatedness/interdependency between data-ownership and multifaceted data-usage requires special attention.
Data, algorithms and anti-trust
Existing anti-trust regulations often block the multi stakeholder cooperation that is a prerequisite for data and algorithm driven innovation. A renewed anti-trust framework should facilitate co-creation and sharing between corporate digital twins, like in lab-settings for Potentially Relevant Data.
Data- and algorithm liability
We need to design a legislative system that links different algorithm impact scenarios with corresponding levels of liability. For instance for (semi) autonomous decision making or man-machine interfaces. In the designing process we should address whether or not private or public characteristics are relevant for liability.
Thought leadership in economics and business
Economics and business are relevant because they influence coordinating mechanisms like markets, organizations and business models. Data and algorithms require an economic and business eco-system that facilitate networked cooperation, personalisation to the max and reverse investment dynamics. This is exactly the opposite of most present day paradigms that are based on efficiency, standardisation and economies of scale.
‘It’s time for Corporate Digital Twin meets SDG’s!’’
We need novel points of view in economics and business to capture the essence of data and algorithms. For the 2020’s we focus on three domains:
Digital Value Creation Model
Every tech-company should have its own Digital Value Creation Model that represent its strategy on how data and algorithms create long term value and impact. By linking input-data with algorithm engineering, and translating their outputs to outcomes and ultimately impact, stakeholders are able to see at a glance what a tech-company’s corporate digital twin is all about.
Value of data
We need to define the value of data on multiple levels. Only when we can measure, price and exchange the value of data, we can take data- and algorithm related decision making to the same level we use to make decisions that involve land, labour and capital. We should also be able to incorporate scarcity and differentiate value for different objectives and/or purposes throughout the entire design, build and use life cycle.
‘Creating successful innovation safe zones for data and algorithm driven innovation is one of the most prominent leadership challenges for the 2020’s.’
Innovation safe zones
To facilitate the exploration focus and seize data and algorithm opportunities to the max we need innovation safe zones. They are specially created hubs where we can pursue networked innovation in lab-settings without being hindered by out-dated concepts like corporate boundaries, existing product portfolio’s or customer loyalty.