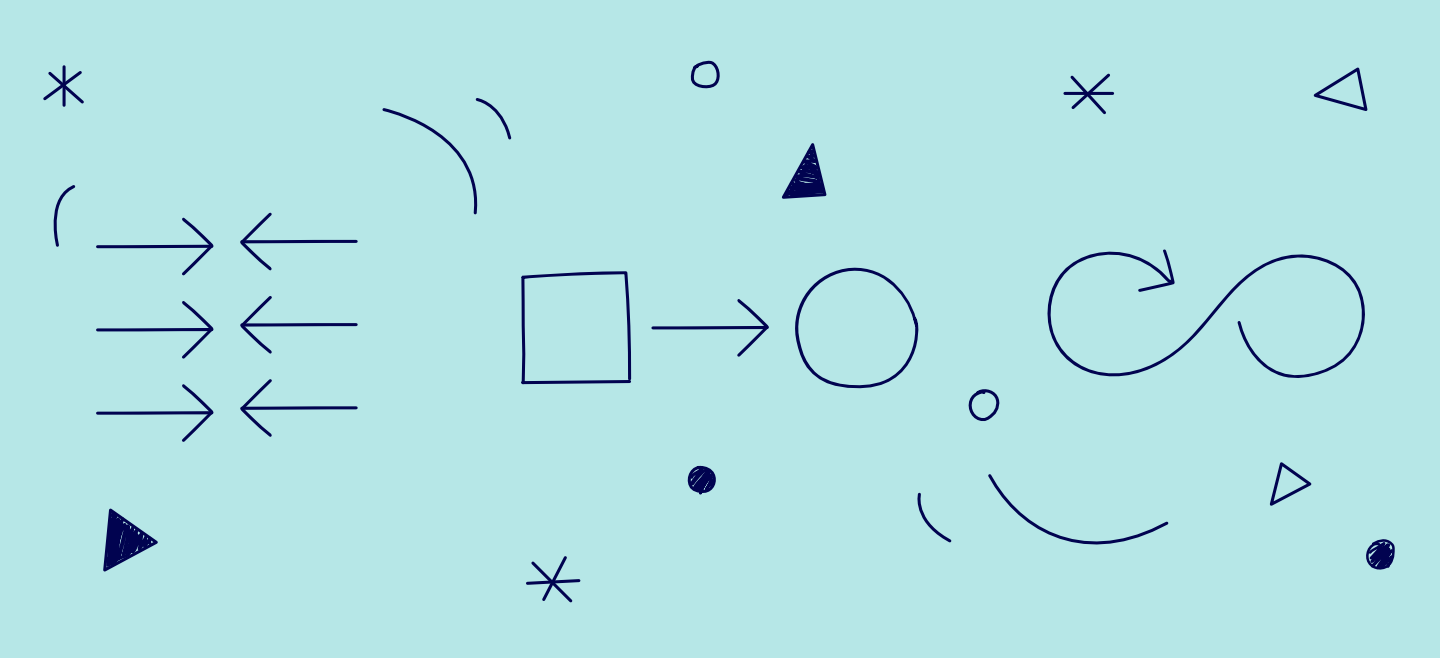
Rethink data: from Big to Relevant
At the beginning of the 2010’s, when data emerged to mainstream it was quickly labelled Big Data. Fuelled by ‘everything is a sensor’ and ‘data is the new gold’ we came to believe that it was all about collecting as much data as possible. Originating from an (out-dated) industrial paradigm ‘bigger, cheaper and faster’ were the leading keywords. As (expensive) technical limitations in sensors, storage capacity, processing speed and interfacing diminished why not collect as much data you could get your hands on?
But as Big Data evolved and the amount of collected data kept growing, so did the number of ‘data-driven disillusions’. For instance we learned that incorporating new data-driven insights in existing work methods can bring uncertainty and ultimately refusal, sharing data requires technical standardization but above all the profound willingness to step back and question strong assumptions about one’s self, and hiring teens with hoodies is no guarantee to avoid writing off large data-related investments when bottom-line results just don’t seem to come through. .
‘Throughout the 2010’s we gradually found out that in many cases more means less. Collecting large amounts of data isn’t the problem. Making this collected data meaningful and profitable is something completely different.’
Introducing (Potentially) Relevant Data
In this manifest we introduce the concept of Relevant Data and (its little cousin) Potentially Relevant Data. We say good-bye to Big Data.
Relevant Data can be defined as data for which it’s generally acknowledged and socially accepted why it is collected, stored and used. In other words, Relevant Data becomes meaningful only in combination with a clear objective and when relevance is beyond any doubt.’.
Think about your phone’s location data that is used to navigate you through traffic on your way home. Or about personal data like age, social background or weight that is valuable for medical reasons. The relevance of these types of data is obvious to you, your network provider, your doctor as well as the medical- or traffic app company you’re familiar with.
‘Since exploring the unknown is the core premise of the data and algorithm revolution, the concept of Relevant Data can only exist in combination with Potentially Relevant Data.’
The uniqueness of Potentially Relevant Data lies in facilitating innovation and experimentation. The add-on ‘Potentially’ refers to a preliminary research status. As soon as relevance is proven beyond any doubt Potentially Relevant Data loses the add-on. In search of the unknown there is now limit to the quantity or diversity of Potentially Relevant Data. However, each research exploration must have an objective (no matter how vague) and a clear definition of its necessity and proportionality.
So again, think about your phone’s location data. For navigation, relevance has been proven. However, your phone’s location data may also hold potential value for other objectives. For instance insurance companies and car manufacturers want to use location data for their acceptance or claim policy. Or anti-terror units have an interest in it to track terrorists. For these and all other objectives your phones location data remains Potentially Relevant Data as long as the exploring stage is on going. Only when all constituencies involved conclude that relevance for a specific objective is beyond any doubt, then it becomes Relevant Data.
Two different operating regimes
Relevant Data and Potentially Relevant Data each have a different operating regime. The dynamics of the interrelatedness between both lead to a process of reflection, framing and re-framing on the meaning of data. It is every company’s own responsibility to design these processes for establishing data relevance in a sense making way. By making this process transparent and by being responsive, companies can show that seizing opportunities and taking responsibilities can go hand in hand.
‘To fight the trust crisis the premier challenge for every tech-company is to design and implement a company specific working method that facilitates the dynamics between Potentially Relevant Data on its way to becoming Relevant Data.’
Relevant Data has an operating regime that aims to maximize the benefits of proven relevance. It is designed to facilitate up scaling, commercialisation or exploitation. This data regime can be characterised as open and supportive to data sharing and co-creation. All who are involved, including data-owner, data-user and data-consumer, agree on how to work with this data, they know its value and limitations, now and in the (near) future.
For Potentially Relevant Data the operating regime is totally different. On the one hand it’s a lab setting with a focus on searching the unknown. The less limitations there are, the better. On the other hand, necessity and proportionality outline its scope and restrictions. As part of researching the potential relevance of data for a specific objective getting rid of non-relevant data as soon as possible is important. This aspect of deleting (non-relevant) data is an important factor in the transition between Potentially Relevant Data becoming Relevant Data.
‘As it seems contrary to the common held belief that more (data) is better, deleting data has long been neglected as a crucial element of sense making related to data and algorithms.’